A 3-step program to provide continuous improvement and better efficiency of EAF technology.
The recent development of smart technology and high-speed computer analysis is rapidly reshaping the traditional Electric Arc Furnace (EAF) process into one that is closely controlled, more predictable and optimized in real-time. The deployment of an array of innovative technologies can enable dynamic process optimization, as well as reduce total energy consumption dramatically.
An example: innovative sensors coupled with computerized data analysis are providing breakthrough solutions to chronic problems such as water leak detection and dynamic process control for each stage of the melting and refining process.
Step 1: Critical Sensors
Implementation of robust sensors is an important first step in establishing a workable EAF Industry 4.0 solution. The sensors provide actual measurements in critical process areas and thereby avoid control errors and inaccuracies that can result when using estimates and assumptions.
Off-gas composition, flow, temperature and pressure provide valuable real-time information necessary for closing a real time EAF mass and energy balance, for optimized control of the quantity and timing of both chemical energy and electrical inputs and for optimized furnace draft control. While reliable off-gas sensors have largely been lacking in the past, Tenova Goodfellow (TGI) has now developed a full suite of commercially robust sensors that provide these critical process measurements.
Step 2: Fundamental Mass & Energy Balance Process Control Models
Typical EAF control is based on kWh/ton. With this conventional method, each heat’s sequencing and energy delivery is essentially the same and is made without consideration for chemical energy inputs, and energy losses and inefficiencies.
A better approach is to control the EAF with real-time mass and energy balances – in this case, the timing and quantity of chemical energy (kWh equivalent) and electrical energy (kWh) is made dynamically on a heat specific basis allowing for the actual total net energy received by the charge after energy losses and process inefficiencies. Tenova’s iEAF ® technology utilizes the critical sensors described above together with a link to the plant’s Level 1 & 2 network to obtain the measurements needed to close real-time EAF mass and energy balances.
Step 3: Digitalization
Tenova’s latest step in developing an Intelligent EAF is an EAF Digitalization Program implemented by a team of data scientists and process experts using cloud data storage technology and machine learning to monitor and analyze EAF process performance, develop improved process control models and dynamically re-tune process models.
Steps 1 and 2 created a digitalized interface within the EAF shop that links operating equipment, critical sensors, Level 1 & 2 networks, and iEAF® process control models to achieve dynamic mass and energy based control of the EAF process. The third step completes the digitalization by leveraging on advanced analytics, machine learning methods and digital cloud services.
Canada
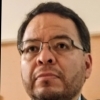
Senior Business Development Manager, Tenova Goodfellow Inc.
Armando Vazquez
Related technologies
Want to know more?
Get in touch with our experts on the subject